CSCO + SPLK - A Financial Engineering Driven Deal (Pt.1)
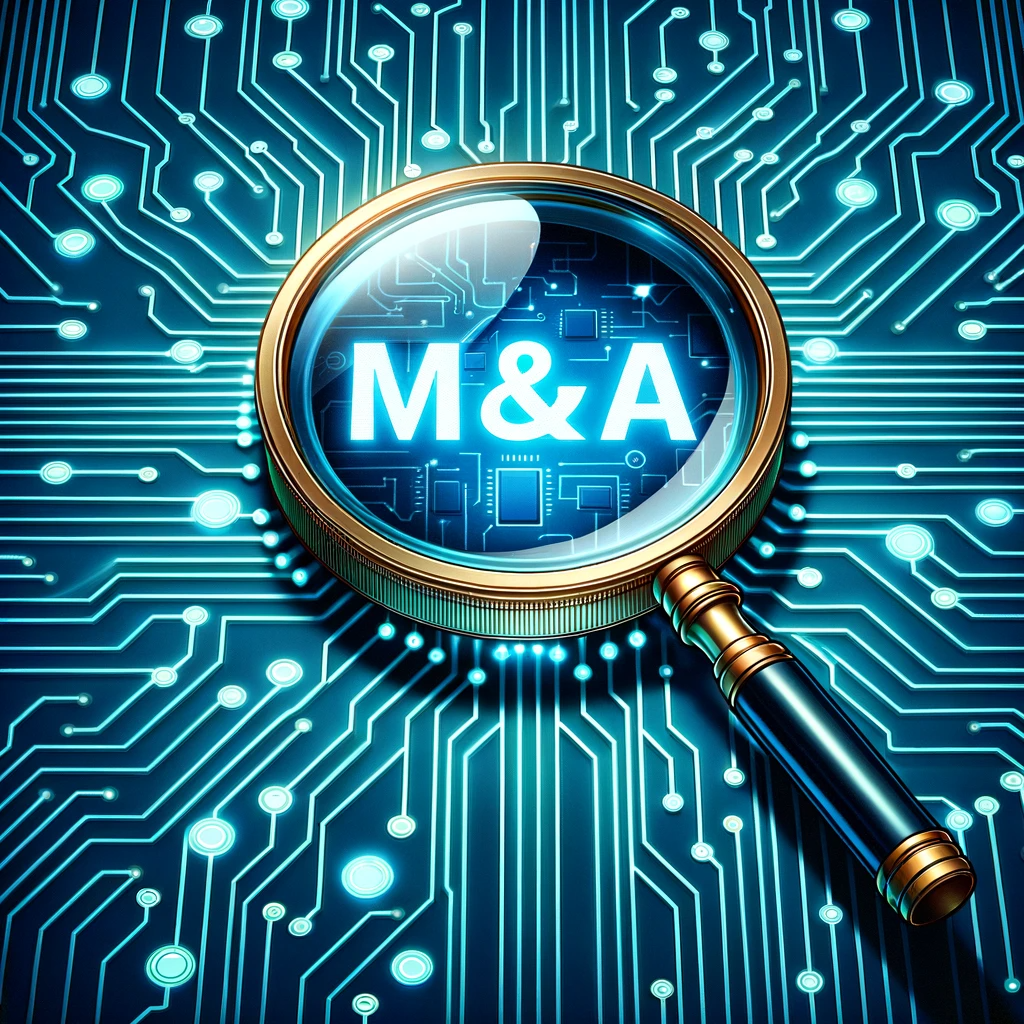
Summary
- SPLK, an old guard in the SIEM market, commands significant market share. However, its legacy architecture is at risk from emerging cloud-native alternatives.
- On the surface, CSCO's reasons for the acquisition may appear unconvincing. Yet, by enhancing operational efficiency, there's potential for unlocking significant shareholder value.
- The market response has been largely pessimistic. While there are clear financial gains available, SPLK's product competitiveness in the long run appears shaky, opening up opportunities for next-gen rivals.
- In Part 1 we discuss why it has been difficult for SPLK to transition to the cloud. In Part 2 we'll cover the arb and investor implications. In Part 3 we'll discuss the competitor implications.
Companies discussed: CSCO, SPLK, PANW, FTNT, DDOG, SNOW, S, CRWD, and Panther Labs.
CSCO's acquisition of SPLK, a deal long rumoured in the industry, is now confirmed. Here's a deep dive into the motivations behind this move, the story of SPLK’s trajectory leading to this acquisition, and what the future could possibly hold for the merged entity.
Deal Financials
- Offer Price: SPLK at $158 per share, giving an equity value of $28bn.
- Net Debt: $1.66bn.
- Closure Timeline: Expected by CY 3Q24.
- Premiums: This deal offers a substantial ~100% premium over the 52-week low and a ~40% premium compared to SPLK's YTD low.
SPLK: Adding some colour and context
Defining SPLK
Founded in 2004, SPLK began its journey focusing on the accumulation and analysis of machine data, often termed as log management. Over time, its role evolved to include saving security log data (which is called SIEM; stands for Security Incident & Event Management), which now constitutes over 70% of its revenue. The remaining revenue streams come from application performance management – a result of collecting application-centric log data and generating business analytics.
The Role of SIEM and its Limitations
Larger enterprises and businesses under stringent regulations heavily rely on SIEMs, such as SPLK's. SIEM providers consolidate security log data in one location, and in the event of a security breach, teams revert to the SIEM to investigate and develop responses. This process is known as incident response and forensics. However, SPLK isn't inherently optimized for broad and efficient use. When juxtaposed with platforms like SNOW, SPLK is far less optimizable for specific use cases, with a cost and complexity that is magnitudes higher. For instance, SNOW has been designed expecting that business analysts will run hundreds of queries per day on PB-scale data. The amount of queries that SPLK's on-prem software needs to serve depends on the customer's threat landscape and how it handles security risks, which varies significantly, meaning some customers only need to execute a small number of queries per day or per week.
The extreme case is a customer that operates in a low-stakes business that hackers have little interest in. Moreover, the company has great security hygiene that facilitates swift risk resolution before they are exploited by hackers and become a security incident. In such a case, the company would rarely use SPLK, maybe only a few times a year. Meanwhile, the company has to procure and provision hardware storage capacity in advance and procure the software license accordingly.
Business Model Evolution
Originally, SPLK’s revenue came from conventional software licenses, beginning with consumption-based licenses based on CPU cores and then shifting the consumption model to the volume of data ingested. It became a necessity for SPLK to address its SIEM's architectural limitations due to 1) the exponential growth of data, 2) the exponential increase in the sophistication and the number of cyber attacks, 3) enterprises' growing adoption of cloud computing, which led to more things requiring monitoring, and 4) due to cloud-native vendors offering SIEMs in a more efficient and scalable way. All of these factors have created enterprise demand for more cost-effective and scalable SIEM solutions.
However, for an on-prem rooted log management vendor, it is not an easy challenge to switch to a cloud-native SaaS solution. We will discuss why in subsequent sections.
Recent Innovations
Like many legacy tech leaders, SPLK hasn't focused on major architectural revamps, but mostly focused on inorganic platform expansion via acquisitions to drive easier TAM and revenue growth. More specifically, the company wants to provide more value-add, not just by storing data, but also helping customers operationalize security data and make it more immediately useful. One notable innovation in recent years by SPLK for supporting its security goals, is introducing automated templates (labelled as SOAR in the following SPLK investor slide). These are designed to activate an automated response to specific incidents. In essence, since SPLK's acquisition of SOAR startup, Phantom, SPLK's subsequent innovations have been around incrementally building out this capability.
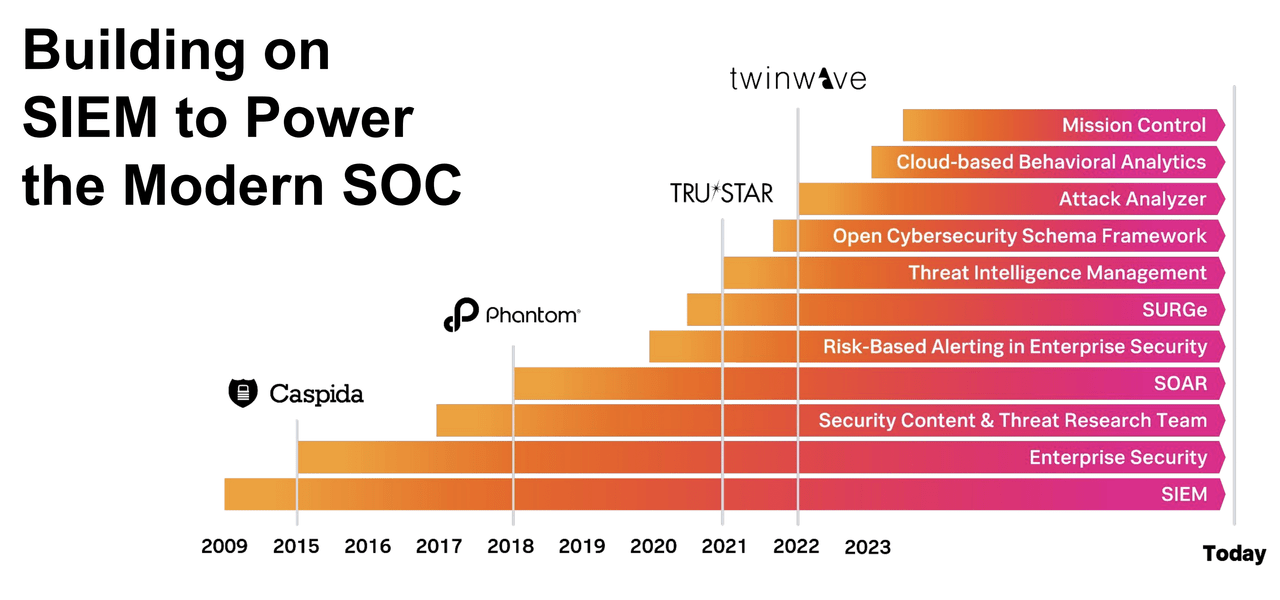
Additionally, SPLK is innovating to enable clients to derive more value from their security data by venturing into observability (i.e., software that collates logs (i.e., any event like a customer login, web page visit, network error, etc.) and monitors a range of metrics across infra, networks, and applications, and conveys the internal state of a system to IT and/or DevOps). Observability is a natural extension as ~50% of use cases within observability are also for security purposes. SPLK is also seeking more non-security use cases for log data.
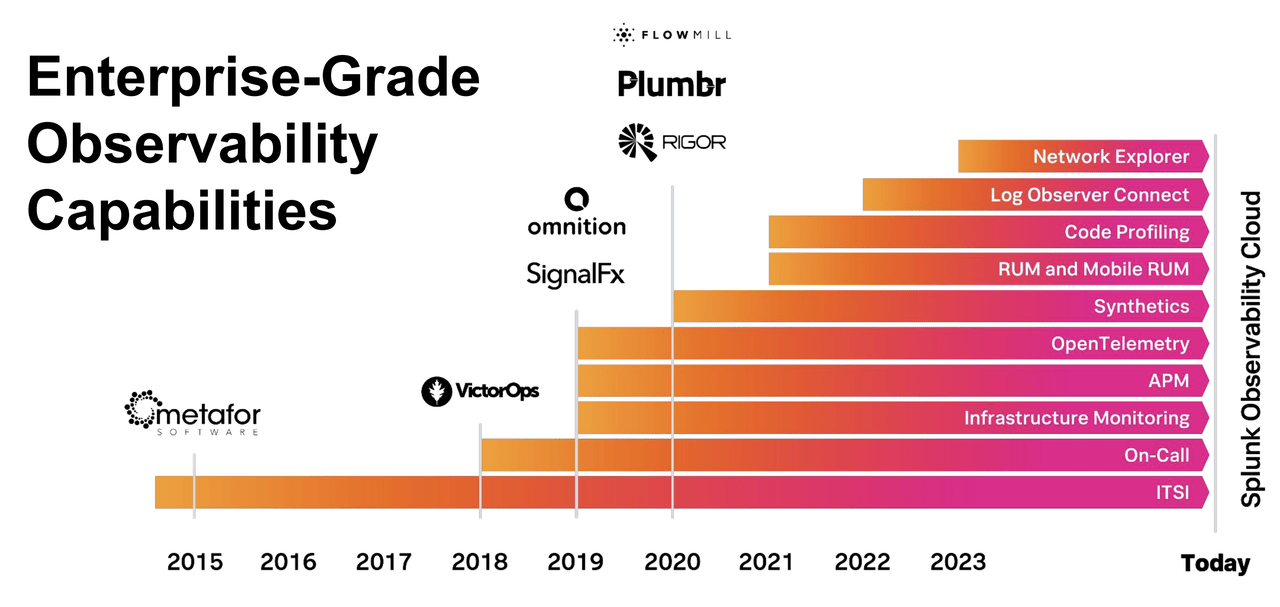
In regards to SPLK's AI potential, SPLK's investor presentation strikes us as illusive and legacy-looking. For available AI features now, only the Splunk AI Assistant is leveraging the new LLM (Large Language Model) technology, while the rest are AI-washing or rebranding SPLK's existing weak-ML-based solution as the new Gen AI feature. Sidenote: for a deep dive into the state of GenAI check out our 2-part series. Generally, the Splunk AI Assistant has two functions:
- A LLM fine-tuned on SPLK's lengthy user documentations to help users more easily navigate them via a natural language interface. This is a worthwhile LLM implementation to help override the poorly documented features that have resulted in high learning costs and poor experiences for users, caused by tons of technical debt accumulated over the company's 20-year history. Though, this is more like a patch over addressing SPLK's weakness rather than a true greenfield GenAI market opportunity.
- A LLM fine-tuned on SPLK's unique querying language, the Search Processing Language (SPL). Similar to many other data solutions before MDS (stands for Modern Data Stack; read Convequity's Modern Data Stack or the SNOW three-part series for a deep dive), SPLK doesn't use standardized SQL that is easier to learn and universally available. It uses proprietary SPL that comes with a steep learning curve, and thus, only a few SOC analysts trained to use SPLK can harness its full potential, further raising the TCO (Total Cost of Ownership). By allowing users to write natural language and get it translated to SPL, SPLK can help reduce its learning gap with standard solutions like SQL (relatedly, SNOW chose to run the industry querying standard of SQL, rather than build proprietary language, which has been a monumental decision for increasing adoption).