Notes - DeepSeek Supply Shock Yet To Come
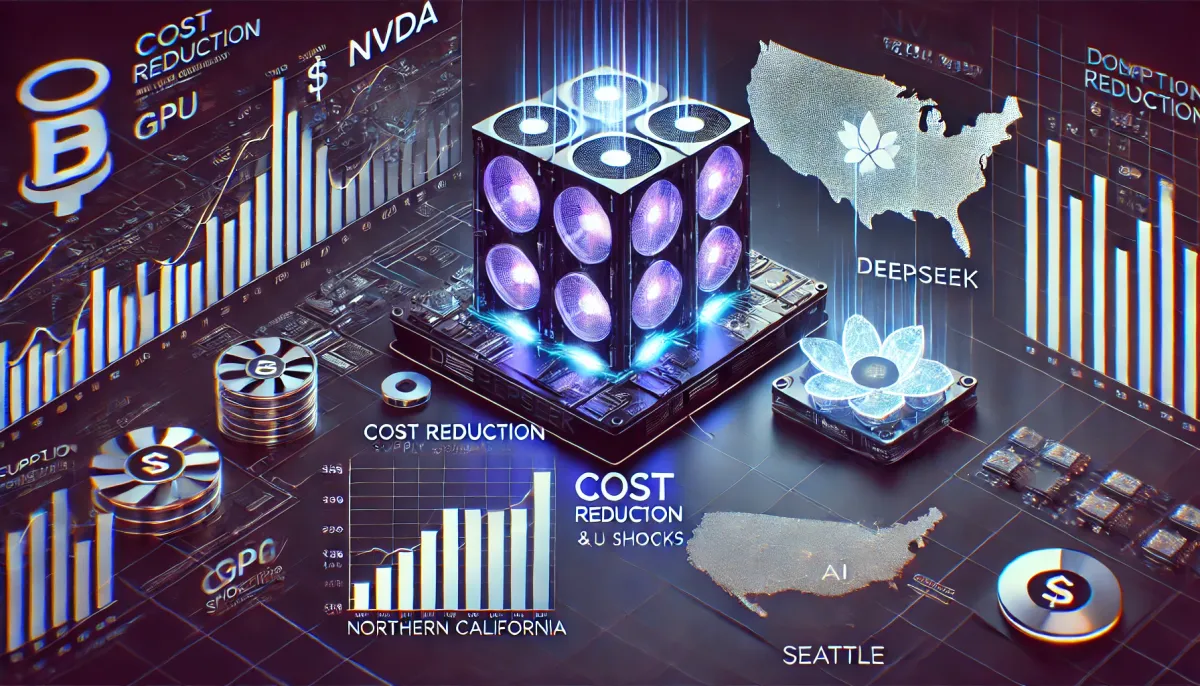
Summary
- DeepSeek introduces a cost-effective training and inference architecture that could reduce AI operational costs to one-tenth, challenging existing infrastructures.
- Recent data shows a short-term spike in AWS H100 spot prices in key tech regions, but this affects only a small fraction of overall GPU demand.
- DeepSeek has potentially triggered a trend that mirrors past IT shifts, where application developers, not infrastructure providers, ultimately drove industry growth.
- This raises questions about NVDA’s ability to maintain its dominance amid a potential shift toward upper-layer value creation as specialized AI ASICs emerge.
DeepSeek is reshaping the US stock market by introducing a dramatically more efficient training and inference architecture, spanning both models and infrastructure. The core thesis is straightforward: if a leading-edge model can be trained and run at just one-tenth of the current cost, the demand for AI chips — and the broader trickle-down spending on infrastructure, models, and related AI investments — declines significantly. Compounding this disruption, CUDA’s dominance is eroding as DeepSeek sidesteps it by leveraging PTX, an assembly language operating just one layer beneath NVIDIA’s CUDA libraries.
At first glance, this may seem counterintuitive. Historically, when emerging technologies see a steep cost reduction before mass adoption, demand often accelerates, more than offsetting the impact of lower prices — a dynamic known as Jevons' Paradox. From mainframes to PCs to mobile devices, this pattern has played out repeatedly. More recently, OpenAI scaled its ARR from near zero to $4bn+ by slashing GPT-4’s operational cost by 12x, further illustrating how AI market dynamics evolve under cost compression.